Network effect
In economics, a network effect (also called network externality or demand-side economies of scale) is the phenomenon by which the value or utility a user derives from a good or service depends on the number of users of compatible products. Network effects are typically positive, resulting in a given user deriving more value from a product as other users join the same network. The adoption of a product by an additional user can be broken into two effects: an increase in the value to all other users ( "total effect") and also the enhancement of other non-users motivation for using the product ("marginal effect").[1]
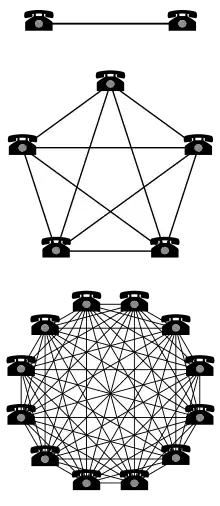
Network effects can be direct or indirect. Direct network effects arise when a given user's utility increases with the number of other users of the same product or technology, meaning that adoption of a product by different users is complementary.[2] This effect is separate from effects related to price, such as a benefit to existing users resulting from price decreases as more users join. Direct network effects can be seen with social networking services, including Twitter, Facebook, Airbnb, Uber, and LinkedIn; telecommunications devices like the telephone; and instant messaging services such as MSN, AIM or QQ.[3] Indirect (or cross-group) network effects arise when there are "at least two different customer groups that are interdependent, and the utility of at least one group grows as the other group(s) grow".[4] For example, hardware may become more valuable to consumers with the growth of compatible software.
Network effects are commonly mistaken for economies of scale, which describe decreasing average production costs in relation to the total volume of units produced. Economies of scale are a common phenomenon in traditional industries such as manufacturing, whereas network effects are most prevalent in new economy industries, particularly information and communication technologies. Network effects are the demand side counterpart of economies of scale, as they function by increasing a customer's willingness to pay due rather than decreasing the supplier's average cost.[5]
Upon reaching critical mass, a bandwagon effect can result. As the network continues to become more valuable with each new adopter, more people are incentivised to adopt, resulting in a positive feedback loop. Multiple equilibria and market tipping are two key potential outcomes in markets that exhibit network effects. Consumer expectations are key in determining which outcomes will result.
Origins
Network effects were a central theme in the arguments of Theodore Vail, the first post-patent president of Bell Telephone, in gaining a monopoly on US telephone services. In 1908, when he presented the concept in Bell's annual report, there were over 4,000 local and regional telephone exchanges, most of which were eventually merged into the Bell System.
Network effects were popularized by Robert Metcalfe, stated as Metcalfe's law. Metcalfe was one of the co-inventors of Ethernet and a co-founder of the company 3Com. In selling the product, Metcalfe argued that customers needed Ethernet cards to grow above a certain critical mass if they were to reap the benefits of their network.[6] According to Metcalfe, the rationale behind the sale of networking cards was that the cost of the network was directly proportional to the number of cards installed, but the value of the network was proportional to the square of the number of users. This was expressed algebraically as having a cost of N, and a value of N2. While the actual numbers behind this proposition were never firm, the concept allowed customers to share access to expensive resources like disk drives and printers, send e-mail, and eventually access the Internet.[7]
The economic theory of the network effect was advanced significantly between 1985 and 1995 by researchers Michael L. Katz, Carl Shapiro, Joseph Farrell, and Garth Saloner.[8] Author, high-tech entrepreneur Rod Beckstrom presented a mathematical model for describing networks that are in a state of positive network effect at BlackHat and Defcon in 2009 and also presented the "inverse network effect" with an economic model for defining it as well.[9] Because of the positive feedback often associated with the network effect, system dynamics can be used as a modelling method to describe the phenomena.[10] Word of mouth and the Bass diffusion model are also potentially applicable.[11]
Adoption and competition
Critical mass
In the early phases of a network technology, incentives to adopt the new technology are low. After a certain number of people have adopted the technology, network effects become significant enough that adoption becomes a dominant strategy. This point is called critical mass. At the critical mass point, the value obtained from the good or service is greater than or equal to the price paid for the good or service.[12]
When a product reaches critical mass, network effects will drive subsequent growth until a stable balance is reached.[13] Therefore, a key business concern must then be how to attract users prior to reaching critical mass. Critical quality is closely related to consumer expectations, which will be affected by price and quality of products or services, the company's reputation and the growth path of the network.[2] Thus, one way is to rely on extrinsic motivation, such as a payment, a fee waiver, or a request for friends to sign up.[14] A more natural strategy is to build a system that has enough value without network effects, at least to early adopters. Then, as the number of users increases, the system becomes even more valuable and is able to attract a wider user base.[15]
Beyond critical mass, the increasing number of subscribers generally cannot continue indefinitely. After a certain point, most networks become either congested or saturated, stopping future uptake. Congestion occurs due to overuse. The applicable analogy is that of a telephone network. While the number of users is below the congestion point, each additional user adds additional value to every other customer. However, at some point, the addition of an extra user exceeds the capacity of the existing system. After this point, each additional user decreases the value obtained by every other user. In practical terms, each additional user increases the total system load, leading to busy signals, the inability to get a dial tone, and poor customer support. Assuming the congestion point is below the potential market size, the next critical point is where the value obtained again equals the price paid. The network will cease to grow at this point if system capacity is not improved. Peer-to-peer (P2P) systems are networks designed to distribute load among their user pool. This theoretically allows P2P networks to scale indefinitely. The P2P based telephony service Skype benefits from this effect and its growth is limited primarily by market saturation.[16]
Market tipping
Network effects give rise to the potential outcome of market tipping, defined as "the tendency of one system to pull away from its rivals in popularity once it has gained an initial edge".[17] Tipping results in a market in which only one good or service dominates and competition is stifled. This is because network effects tend to incentivise users to coordinate their adoption of a single product. Therefore, tipping can result in a natural form of market concentration in markets that display network effects.[18] However, the presence of network effects does not necessarily imply that a market will tip; the following additional conditions must be met:
- The utility derived by users from network effects must exceed the utility they derive from differentiation
- Users must have high costs of multihoming (i.e. adopting more than one competing networks)
- Users must have high switching costs
If any of these three conditions are not satisfied, the market may fail to tip and multiple products with significant market shares may coexist.[4] One such example is the U.S. instant messaging market, which remained an oligopoly despite significant network effects. This can be attributed to the low multi-homing and switching costs faced by users.
Market tipping does not imply permanent success in a given market. Competition can be reintroduced into the market due to shocks such as the development of new technologies. Additionally, if the price is raised above customers' willingness to pay, this may reverse market tipping.[4]
Multiple equilibria and expectations
Networks effects often result in multiple potential market equilibrium outcomes. The key determinant in which equilibrium will manifest are the expectations of the market participants, which are self-fulfilling.[2] Because users are incentivised to coordinate their adoption, user will tend to adopt the product that they expect to draw the largest number of users. These expectations may be shaped by path dependence, such as a perceived first-mover advantage, which can result in lock-in. The most commonly cited example of path dependence is the QWERTY keyboard, which owes its ubiquity to its establishment of an early lead in the keyboard layout industry and high switching costs, rather than any inherent advantage over competitors. Other key influences of adoption expectations can be reputational (e.g. a firm that has previously produced high quality products may be favoured over a new firm).[19]
Markets with network effects may result in inefficient equilibrium outcomes. With simultaneous adoption, users may fail to coordinate towards a single agreed upon product, resulting in ‘splintering’ among different networks, or may coordinate to lock-in to a different product than the one that is best for them.[2]
Technology lifecycle
If some existing technology or company whose benefits are largely based on network effects starts to lose market share against a challenger such as a disruptive technology or open standards based competition, the benefits of network effects will reduce for the incumbent, and increase for the challenger. In this model, a tipping point is eventually reached at which the network effects of the challenger dominate those of the former incumbent, and the incumbent is forced into an accelerating decline, whilst the challenger takes over the incumbent's former position.[20]
Sony's Betamax and Victor Company of Japan (JVC)'s video home system (VHS) can both be used for video cassette recorders (VCR), but the two technologies are not compatible. Therefore, the VCR that is suitable for one type of cassette cannot fit in another. VHS's technology gradually surpassed Betamax in the competition. In the end, Betamax lost its original market share and was replaced by VHS.[21]
Negative network externalities
Negative network externalities, in the mathematical sense, are those that have a negative effect compared to normal (positive) network effects. Just as positive network externalities (network effects) cause positive feedback and exponential growth, negative network externalities create negative feedback and exponential decay. In nature, negative network externalities are the forces that pull towards equilibrium, are responsible for stability, and represent physical limitations keeping systems bounded.
Besides, Negative network externalities has four characteristics, which are namely, more login retries, longer query times, longer download times and more download attempts.[22] Therefore, congestion occurs when the efficiency of a network decreases as more people use it, and this reduces the value to people already using it. Traffic congestion that overloads the freeway and network congestion on connections with limited bandwidth both display negative network externalities.[23]
Braess' paradox suggests that adding paths through a network can have a negative effect on performance of the network.[24]
Interoperability
Interoperability has the effect of making the network bigger and thus increases the external value of the network to consumers. Interoperability achieves this primarily by increasing potential connections and secondarily by attracting new participants to the network. Other benefits of interoperability include reduced uncertainty, reduced lock-in, commoditization and competition based on price.[25]
Interoperability can be achieved through standardization or other cooperation. Companies involved in fostering interoperability face a tension between cooperating with their competitors to grow the potential market for products and competing for market share.[26]
Compatibility and incompatibility
Product compatibility is closely related to network externalities in company's competition, which refers to two systems that can be operated together without changing. Compatible products are characterized by better matching with customers, so they can enjoy all the benefits of the network without having to purchase products from the same company. However, not only products of compatibility will intensify competition between companies, this will make users who had purchased products lose their advantages, but also proprietary networks may raise the industry entry standards. Compared to large companies with better reputation or strength, weaker companies or small networks will more inclined to choose compatible products.[27]
Besides, the compatibility of products is conducive to the company's increase in market share. For example, the Windows system is famous for its operating compatibility, thereby satisfying consumers' diversification of other applications. As the supplier of Windows systems, Microsoft benefits from indirect network effects, which cause the growing of the company's market share.[28]
Incompatibility is the opposite of compatibility. Because incompatibility of products will aggravate market segmentation and reduce efficiency, and also harm consumer interests and enhance competition. The result of the competition between incompatible networks depends on the complete sequential of adoption and the early preferences of the adopters.[29] Effective competition determines the market share of companies, which is historically important.[30] Since the installed base can directly bring more network profit and increase the consumers' expectations, which will have a positive impact on the smooth implementation of subsequent network effects.
Open versus closed standards
In communication and information technologies, open standards and interfaces are often developed through the participation of multiple companies and are usually perceived to provide mutual benefit. But, in cases in which the relevant communication protocols or interfaces are closed standards, the network effect can give the company controlling those standards monopoly power. The Microsoft corporation is widely seen by computer professionals as maintaining its monopoly through these means. One observed method Microsoft uses to put the network effect to its advantage is called Embrace, extend and extinguish.[31]
Mirabilis is an Israeli start-up which pioneered instant messaging (IM) and was bought by America Online. By giving away their ICQ product for free and preventing interoperability between their client software and other products, they were able to temporarily dominate the market for instant messaging. The IM technology has completed the use from the home to the workplace, because of its faster processing speed and simplified process characteristics. Because of the network effect, new IM users gained much more value by choosing to use the Mirabilis system (and join its large network of users) than they would use a competing system. As was typical for that era, the company never made any attempt to generate profits from its dominant position before selling the company.[32]
Examples
Financial exchanges
Stock exchanges and derivatives exchanges feature a network effect. Market liquidity is a major determinant of transaction cost in the sale or purchase of a security, as a bid–ask spread exists between the price at which a purchase can be made versus the price at which the sale of the same security can be made. As the number of sellers and buyers in the exchange, who have the symmetric information increases, liquidity increases, and transaction costs decrease.[33] This then attracts a larger number of buyers and sellers to the exchange.
The network advantage of financial exchanges is apparent in the difficulty that startup exchanges have in dislodging a dominant exchange. For example, the Chicago Board of Trade has retained overwhelming dominance of trading in US Treasury bond futures despite the startup of Eurex US trading of identical futures contracts. Similarly, the Chicago Mercantile Exchange has maintained dominance in trading of Eurobond interest rate futures despite a challenge from Euronext.Liffe.
Cryptocurrencies
Cryptocurrencies like Bitcoin also features network effects. Bitcoin's unique properties make it an attractive asset to users and investors, and as more users join the network, the more secure it gets. When the network and community grows, a network effect can occur, making it more likely that new people will join. Also, Bitcoin tends to become financially more valuable as more people invest in it, which may lead to more investors doing the same.[34]
Software
The widely used computer software benefits from powerful network effects. The software-purchase characteristic is that it is easily influenced by the opinions of others, so the customer base of the software is the key to realizing a positive network effect. Although customers’ motivation for choosing software is related to the product itself, media interaction and word-of-mouth recommendations from purchased customers can still increase the possibility of software being applied to other customers who have not purchased it, thereby resulting in network effects.[35]
In 2007 Apple released the iPhone followed by the app store. Most iPhone apps rely heavily on the existence of strong network effects. This enables the software to grow in popularity very quickly and spread to a large userbase with very limited marketing needed. The Freemium business model has evolved to take advantage of these network effects by releasing a free version that will not limit the adoption or any users and then charge for premium features as the primary source of revenue. Furthermore, some software companies will launch free trial versions during the trial period to attract buyers and reduce their uncertainty. The duration of free time is related to the network effect. The more positive feedback the company received, the shorter the free trial time will be.[36]
Web sites
Many web sites benefit from a network effect. One example is web marketplaces and exchanges. For example, eBay would not be a particularly useful site if auctions were not competitive. As the number of users grows on eBay, auctions grow more competitive, pushing up the prices of bids on items. This makes it more worthwhile to sell on eBay and brings more sellers onto eBay, which, in turn, drives prices down again due to increased supply. Increased supply brings even more buyers to eBay. Essentially, as the number of users of eBay grows, prices fall and supply increases, and more and more people find the site to be useful.
Network effects were used as justification in business models by some of the dot-com companies in the late 1990s. These firms operated under the belief that when a new market comes into being which contains strong network effects, firms should care more about growing their market share than about becoming profitable. The justification was that market share would determine which firm could set technical and marketing standards and giving these companies a first-mover advantage.[37]
Social networking websites are good examples. The more people register onto a social networking website, the more useful the website is to its registrants.[38]
Google uses the network effect in its advertising business with its Google AdSense service. AdSense places ads on many small sites, such as blogs, using Google technology to determine which ads are relevant to which blogs. Thus, the service appears to aim to serve as an exchange (or ad network) for matching many advertisers with many small sites. In general, the more blogs AdSense can reach, the more advertisers it will attract, making it the most attractive option for more blogs.
By contrast, the value of a news site is primarily proportional to the quality of the articles, not to the number of other people using the site. Similarly, the first generation of search engines experienced little network effect, as the value of the site was based on the value of the search results. This allowed Google to win users away from Yahoo! without much trouble, once users believed that Google's search results were superior. Some commentators mistook the value of the Yahoo! brand (which does increase as more people know of it) for a network effect protecting its advertising business.
Rail gauge
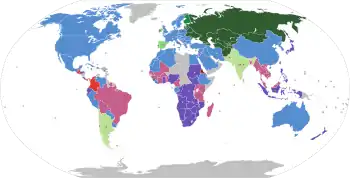
There are strong network effects in the initial choice of rail gauge, and in gauge conversion decisions. Even when placing isolated rails not connected to any other lines, track layers usually choose a standard rail gauge so they can use off-the-shelf rolling stock. Although a few manufacturers make rolling stock that can adjust to different rail gauges, most manufacturers make rolling stock that only works with one of the standard rail gauges.
Credit cards
For credit cards that are now widely used, large-scale applications on the market are closely related to network effects. Credit card, as one of the currency payment methods in the current economy,[39] which was originated in 1949. Early research on the circulation of credit cards at the retail level found that credit card interest rates were not affected by macroeconomic interest rates and remained almost unchanged. Later, credit cards gradually entered the network level due to changes in policy priorities and became a popular trend in payment in the 1980s.[37] Different levels of credit cards separate benefit from two types of network effects. The application of credit cards related to external network effects, which is because when this has become a payment method, and more people use credit cards. Each additional person uses the same credit card, the value of rest people who use the credit card will increase.[21] Besides, the credit card system at the network level could be seen as a two-sided market. On the one hand, the number of cardholders attracts merchants to use credit cards as a payment method. On the other hand, an increasing number of merchants can also attract more new cardholders. In other words, the use of credit cards has increased significantly among merchants which leads to increased value. This can conversely increase the cardholder's credit card value and the number of users. Moreover, credit card services also display a network effect between merchant discounts and credit accessibility. When credit accessibility increases which greater sales can be obtained, merchants are willing to be charged more discounts by credit card issuers.[40]
Visa has become a leader in the electronic payment industry through the network effect of credit cards as its competitive advantage. Till 2016, Visa's credit card market share has risen from a quarter to as much as half in four years. Visa is benefit from the network effect. Since every additional Visa cardholder is more attractive to merchants, and merchants can also attract more new cardholders through the brand. In other words, the popularity and convenience of Visa in the electronic payment market, lead more people and merchants choose to use Visa, which greatly increases the value of Visa.[41]
See also
- Anti-competitive practices
- Anti-rival good
- Beckstrom's law
- Betamax
- Business cluster
- Economies of density
- First-mover advantage
- Market failure
- Metcalfe's law
- Monopoly
- Monopsony
- Oligopoly
- Open format
- Open system (computing)
- Path dependence
- Reed's law
- Returns to scale (increasing returns)
- Social multiplier effect
- Two-sided market
References
- Shapiro, Carl. (1999). Information rules : a strategic guide to the network economy. Varian, Hal R. Boston, Mass.: Harvard Business School Press. ISBN 0-87584-863-X. OCLC 39210116.
- The new Palgrave dictionary of economics. Jones, Garett (Third ed.). London. ISBN 978-1-349-95189-5. OCLC 1029103812.CS1 maint: others (link)
- Klemperer, P. (2018). The New Palgrave Dictionary of Economics. London: Macmillan Publishers Ltd.
- Hagiui, Andrei (2018). The Palgrave Encyclopedia of Strategic Management. Cambridge, MA: Macmillan Publishers Ltd. pp. 1104–1107.
- The new Palgrave dictionary of economics (Living ed.). London. ISBN 978-1-349-95121-5. OCLC 1111663693.
- "It's All In Your Head". Forbes. 2007-05-07. Retrieved 2010-12-10.
- Madureira, António; den Hartog, Frank; Bouwman, Harry; Baken, Nico (2013). "Empirical validation of Metcalfe's law: How Internet usage patterns have changed over time". Information Economics and Policy. 25 (4): 246–256. doi:10.1016/j.infoecopol.2013.07.002.
- Knut Blind (2004). The economics of standards: theory, evidence, policy. Edward Elgar Publishing. ISBN 978-1-84376-793-0.
- Buley, Taylor (2009-07-31). "How To Value Your Networks". Forbes. Retrieved 2010-12-10.
- Kumar, Ravi (2018-07-30). "Understanding the basics of Network Effects — The Power of the Platform". Medium. Retrieved 2020-10-30.
- Jorgenson, Eric (2020-05-06). "The Power of Network Effects: Why they make such Valuable Companies, and how to Harness them". Medium. Retrieved 2020-10-30.
- Grajek, Michał; Kretschmer, Tobias (2012-11-01). "Identifying critical mass in the global cellular telephony market". International Journal of Industrial Organization. 30 (6): 496–507. doi:10.1016/j.ijindorg.2012.06.003. ISSN 0167-7187.
- Evans, David S.; Schmalensee, Richard (2010-01-03). "Failure to Launch: Critical Mass in Platform Businesses". Review of Network Economics. 9 (4). doi:10.2202/1446-9022.1256. hdl:1721.1/76685. ISSN 1446-9022. S2CID 201056684.
- Sledgianowsk i, Deb; Kulviwat, Songpol (2009-06-01). "Using Social Network Sites: The Effects of Playfulness, Critical Mass and Trust in a Hedonic Context". The Journal of Computer Information Systems. 49 (4): 74–83. doi:10.1080/08874417.2009.11645342 (inactive 2021-01-14). eISSN 2380-2057. ISSN 0887-4417.CS1 maint: DOI inactive as of January 2021 (link)
- Lu, Yueming; Wu, Xu; Zhang, Xi (eds.). Trustworthy computing and services : International Conference, ISCTCS 2014, Beijing, China, November 28-29, 2014, Revised selected papers. Heidelberg. ISBN 978-3-662-47401-3. OCLC 911938121.
- Gunduz, Gurhan; Yuksel, Murat (2016-05-08). "Popularity-based scalable peer-to-peer topology growth". Computer Networks. 100: 124–140. doi:10.1016/j.comnet.2016.02.017. ISSN 1389-1286.
- Katz, Michael L.; Shapiro, Carl (June 1994). "Systems Competition and Network Effects". Journal of Economic Perspectives. 8 (2): 93–115. doi:10.1257/jep.8.2.93. ISSN 0895-3309.
- 1. Shapiro 2. Varian, 1. Carl 2. Hal (1998). Information Rules. Boston: Harvard Business School Press.
- Robert M. Grant (2009). Contemporary Strategy Analysis. John Wiley & Sons. ISBN 978-0-470-74710-0.
- Wolf, James R.; Portegys, Thomas E. (September 2007). "Technology Adoption in the Presence of Network Externalities: A Web-Based Classroom Game". INFORMS Transactions on Education. 8 (1): 49–54. doi:10.1287/ited.8.1.49. ISSN 1532-0545. S2CID 8736463.
- "Unit 21 Innovation, information, and the networked economy". www.core-econ.org. Retrieved 2020-10-30.
- Asvanund, Atip; Clay, Karen; Krishnan, Ramayya; Smith, Michael D. (June 2004). "An Empirical Analysis of Network Externalities in Peer-to-Peer Music-Sharing Networks". Information Systems Research. 15 (2): 155–174. doi:10.1287/isre.1040.0020. ISSN 1047-7047.
- Zhang, Mengyuan; Yang, Lei; Gong, Xiaowen; He, Shibo; Zhang, Junshan (August 2018). "Wireless Service Pricing Competition Under Network Effect, Congestion Effect, and Bounded Rationality". IEEE Transactions on Vehicular Technology. 67 (8): 7497–7507. doi:10.1109/TVT.2018.2822843. ISSN 0018-9545. S2CID 52003270.
- Lin, Henry; Roughgarden, Tim; Tardos, Éva; Walkover, Asher. "Stronger Bounds on Braess's Paradox and the Maximum Latency of Selfish Routing" (PDF). Stanford Theory. Society for Industrial and Applied Mathematics. Retrieved 16 September 2014.
- Carl Shapiro and Hal R. Varian (1999). Information Rules. Harvard Business School Press. p. 229. ISBN 0-87584-863-X.
- Carl Shapiro and Hal R. Varian (1999). Information Rules. Harvard Business School Press. p. 227. ISBN 0-87584-863-X.
- Katz, Michael; Shapiro, Carl (1985-06-01). "Network Externalities, Competition, and Compatibility". The American Economic Review. 75 (3): 424–440. eISSN 1944-7981. ISSN 0002-8282.
- Wu, Jing; Li, He; Lin, Zhangxi; Zheng, Haichao (September 2017). "Competition in wearable device market: the effect of network externality and product compatibility". Electronic Commerce Research. 17 (3): 335–359. doi:10.1007/s10660-016-9227-6. ISSN 1389-5753. S2CID 45293373.
- Farrell, Joseph; Saloner, Garth (1986). "Installed Base and Compatibility: Innovation, Product Preannouncements, and Predation". The American Economic Review. 76 (5): 940–955. ISSN 0002-8282. JSTOR 1816461.
- Farrell, Joseph; Klemperer, Paul (2007), "Chapter 31 Coordination and Lock-In: Competition with Switching Costs and Network Effects", Handbook of Industrial Organization, Elsevier, 3, pp. 1967–2072, doi:10.1016/s1573-448x(06)03031-7, ISBN 978-0-444-82435-6, retrieved 2020-10-31
- "US Department of Justice Proposed Findings of Fact". Usdoj.gov. Retrieved 2016-04-28.
- Chordas, Lori; Thompson, Erick; Vondrick, Glen (2003-07-01). "Instant connection". Best's Review. Oldwick: A.M. Best Company. 104 (3): 100. eISSN 2161-282X. ISSN 1527-5914.
- Nel, George F.; Smit, Eon; Brummer, Leon M. (2018-04-12). "The link between Internet investor relations and information asymmetry". South African Journal of Economic and Management Sciences. 21 (1). doi:10.4102/sajems.v21i1.1966. ISSN 2222-3436.
- "What Is a Network Effect?". Binance Academy. Retrieved 2021-01-04.
- Kemper, Andreas. (2010). Valuation of network effects in software markets : a complex networks approach. Heidelberg: Physica. ISBN 978-3-7908-2367-7. OCLC 697300408.
- Cheng, Hsing Kenneth; Liu, Yipeng (2012). "Optimal Software Free Trial Strategy: The Impact of Network Externalities and Consumer Uncertainty". Information Systems Research. 23 (2): 488–504. doi:10.1287/isre.1110.0348. ISSN 1047-7047. JSTOR 23274435.
- Jones, Garett. The new Palgrave dictionary of economics (Third ed.). London. ISBN 978-1-349-95189-5. OCLC 1029103812.
- Belvaux, Bertrand (2011). "The Development of Social Media: Proposal for a Diffusion Model Incorporating Network Externalities in a Competitive Environment". Recherche et Applications en Marketing - English Version. 26 (3): 7–22. doi:10.1177/205157071102600301. S2CID 168202506.
- Shy, Oz (2001). The economics of network industries s. Cambridge, U.K.: Cambridge University Press. ISBN 0-511-03224-2. OCLC 559215717.
- Chakravorti, Sujit; To, Ted (2007-06-01). "A theory of credit cards". International Journal of Industrial Organization. 25 (3): 583–595. doi:10.1016/j.ijindorg.2006.06.005. ISSN 0167-7187.
- "How Visa Created a Network Effect". Market Realist. Retrieved 2020-10-30.
External links
- Coordination and Lock-In: Competition with Switching Costs and Network Effects, Joseph Farrell and Paul Klemperer.
- Network Externalities (Effects), S. J. Liebowitz, Stephen E. Margolis.
- An Overview of Network Effects, Arun Sundararajan.
- The Economics of Networks, Nicholas Economides.